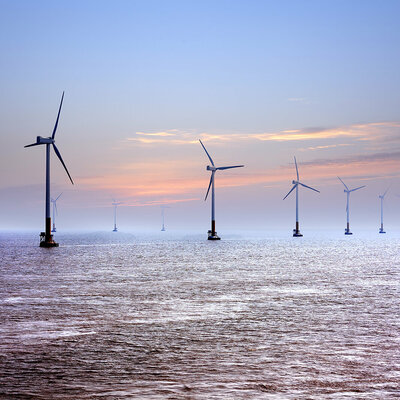
The integration of distributed and supply-dependent primary energies represents a major challenge for the transformation of energy systems. Modern artificial intelligence and machine learning technologies make a significant contribution to meeting this challenge in many areas: in the semi-automatic management of electricity grids, in the insight-driven marketing of decentralised energy systems, in the forecasting of load and generation time series, to name but a few. The close linking of energy systems and ICT infrastructure in smart grids also requires an adaptive and autonomous "immune system" in order to deal with attacks against the infrastructure and failures of subsystems.
The Power Systems Intelligence Group therefore researches and develops solutions at the interface between the power grid, the energy market, artificial intelligence and a cyber-resilient system understanding. Our vision: the AI-empowered Smart Grid.
Every autonomous, pro-active system needs a model of its environment and a prediction of future system states. This can be the active power input of a wind or photovoltaic park, the demand in energetic neighbourhoods, the available flexibility for the provision of system services or the price development of the energy market. The digitalization of the energy supply opens up data volumes that represent a suitable basis for machine learning. In order to draw meaningful conclusions from these large amounts of data and to train systems for autonomous operation, approaches from deep learning have established themselves as a worthwhile research subject with impressive results. Structures such as deep recurrent networks are suitable for predicting time series or market behaviour. They also allow any systems to be simulated and can independently model complex issues using reinforcement learning. This technique, known as a surrogate model, makes it possible to simulate connections in the power grid, derive and correct sensor values or determine operating parameters for network management such as reactive power compensation factors almost in real time.
Our research in the field of machine learning therefore aims to contribute domain knowledge about the power grid and the energy market in the form of architectures for artificial neural networks to artificial intelligence and to make use of the methods of deep learning to enable prototypes for cyber-resilient network management and economic, intelligent action in energy markets. We work closely with the Computational Intelligence Department at the Carl von Ossietzky University of Oldenburg and play a key role in shaping the Deep Learning Competence Cluster.
Increasing decentralisation of energy supply is leading to increasing decentralisation of control and monitoring intelligence. We take the spatial and topological distribution of system components into account by developing distributed, (partially) autonomous agent systems. We work closely with the Department of Energy Informatics at Leibniz Universität Hannover and the OFFIS group Simulation and Agents in Multiple Domains. Our focus is on upgrading individual agents, who learn operating and trading strategies independently and bring the flexibility of the components they represent optimally into the overall system. The issues of network stability and the provision of regional system services also play a key role in this context. Learning agents turn decentralised energy systems into valuable, pro-active assets for distribution network operation. This contributes to a reliable and efficient energy supply and contributes to mastering the increasing complexity of the overall system. Furthermore, the detection of misconduct and other anomalies in power and communication networks is another part of our work.
Our research in the field of distributed and learning systems therefore aims at learning network and system characteristics and AI-supported analysis of possible attack scenarios. The unique research field of Adversarial Resilience Learning enables us to make the power grid a self-adapting, secure and cyber-resilient overall system, even in the case of strong digitalization. This goal connects us with the Automation, Communication and Control group, which researches the stable and reliable operation of dynamic systems.
Wolgast, Thomas and Wenninghoff, Nils and Balduin, Stephan and Veith, Eric and Fraune, Bastian and Woltjen, Torben and Nieße, Astrid; SoftwareX; 04 / 2023
Veith, Eric and Wellßow, Arlena and Uslar, Mathias; Frontiers in Energy Research; 2023
Balduin, Stephan and Veith, Eric M. S. P. and Lehnhoff, Sebastian; Simulation and Modeling Methodologies, Technologies and Applications; 2023
Alexander Berezin, Stephan Balduin, Thomas Oberließen, Eric Veith, Sebastian Peter, and Sebastian Lehnhoff; International Journal of Electrical and Electronic Engineering & Telecommunications; 2022
Wolgast, Thomas and Ferenz, Stephan and Nieße, Astrid; IEEE Access; 01 / 2022
Stephan Balduin. and Eric Veith. and Sebastian Lehnhoff.; Proceedings of the 12th International Conference on Simulation and Modeling Methodologies, Technologies and Applications - SIMULTECH,; 2022
Gerster, Johannes and Sarstedt, Marcel and Veith, Eric M. S. P. and Lehnhoff, Sebastian and Hofmann, Lutz; 2021 IEEE PES Innovative Smart Grid Technologies Europe (ISGT-Europe); 2021
Johannes Gerster and Marcel Sarstedt and Eric M. S. P. Veith and Sebastian Lehnhoff and Lutz Hofmann; ENERGY 2021, The Eleventh International Conference on Smart Grids, Green Communications and IT Energy-aware Technologies; May / 2021
Sarstedt, Marcel and Kluß, Leonard and Gerster, Johannes and Meldau, Tobias and Hofmann, Lutz; Energies; 2021
Balduin, Stephan and Veith, Eric M. S. P. and Berezin, Alexander and Lehnhoff, Sebastian and Oberließen, Thomas and Kittl, Chris and Hiry, Johannes and Rehtanz, Christian and Torres-Villareal, Giancarlo and Leksawat, Sasiphon and Kubis, Andreas and Frankenbach, Marc-Aurel; 2021 IEEE PES Innovative Smart Grid Technologies Europe (ISGT-Europe); 2021